The Importance of Healthcare Datasets for Machine Learning
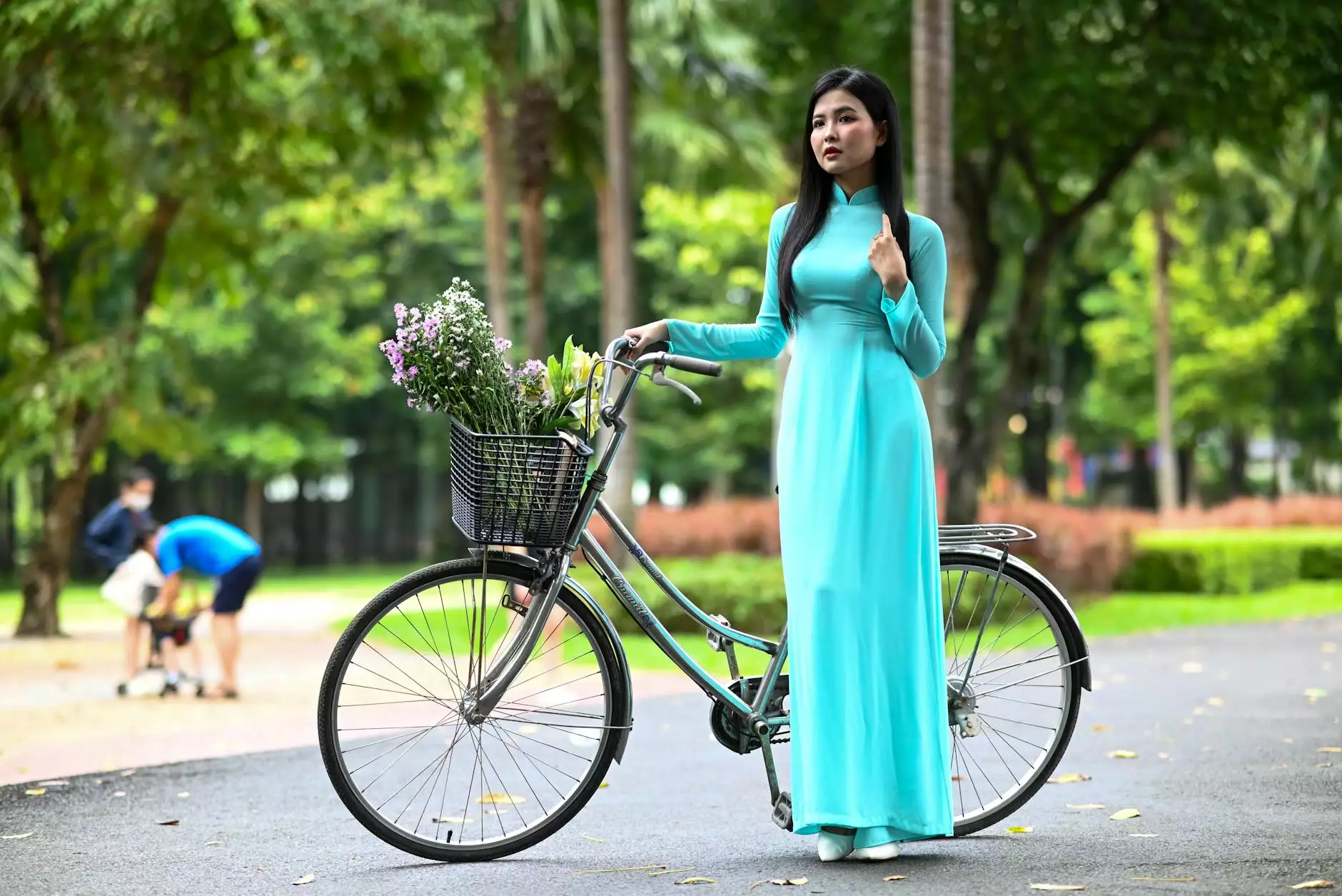
In the rapidly evolving digital age, the intersection of technology and healthcare has opened up fascinating opportunities, particularly through the utilization of healthcare datasets for machine learning. This integration is revolutionizing the way healthcare providers, researchers, and technologists approach patient care, medical research, and operational efficiency.
Understanding Healthcare Datasets
Before delving into their role in machine learning, it's crucial to understand what constitutes a healthcare dataset. These datasets refer to collections of patient data, clinical records, treatment responses, demographic information, and much more. They can originate from various sources, including:
- Electronic Health Records (EHRs): Detailed patient records used by clinicians.
- Health Surveys: Population-level data gathered through surveys pertaining to health behaviors and conditions.
- Clinical Trials: Datasets generated from trials testing new drugs and therapies.
- Wearable Health Devices: Data collected from sensors and devices used in monitoring patient health.
- Public Health Databases: Government and organization-maintained data banks related to health trends and statistics.
The Role of Machine Learning in Healthcare
Machine learning, a subset of artificial intelligence, enables systems to learn from data patterns and improve over time without explicit programming. In healthcare, this capability can enhance various aspects, including:
- Predictive Analytics: Predicting disease outbreaks and patient admissions.
- Personalized Medicine: Tailoring treatment plans based on individual genetic makeup and medical history.
- Automated Diagnostics: Utilizing algorithms to diagnose conditions from medical images.
- Operational Efficiency: Streamlining administrative processes to reduce costs.
- Patient Monitoring: Continuous monitoring of patients through wearable devices and apps.
Connecting Machine Learning with Healthcare Datasets
Integrating healthcare datasets for machine learning is not just beneficial but essential for creating accurate models. Here’s a deeper look into how these datasets fuel machine learning advancements:
1. Enhancing Data Quality and Diversity
Machine learning models thrive on diverse and high-quality data. In healthcare, this means aggregating datasets that encompass various demographics, health conditions, and treatment responses. A rich dataset empowers algorithms to recognize patterns across different populations, paving the way for more inclusive and effective healthcare solutions.
2. Fueling Innovation in Research
Healthcare datasets have become a treasure trove for researchers looking to identify new treatment pathways or understand disease mechanisms. With machine learning, researchers can analyze vast amounts of data quickly, uncovering insights that would be nearly impossible through traditional analytical methods.
3. Improving Diagnostic Accuracy
When machine learning algorithms are trained on extensive and relevant healthcare datasets, they have the potential to outperform human clinicians in diagnosing conditions. AI-assisted diagnostic tools can analyze medical images, lab results, and patient history to provide recommendations or second opinions, ultimately enhancing patient outcomes.
Challenges and Ethical Considerations
While the integration of healthcare datasets for machine learning brings transformative potential, it also presents several challenges:
- Data Privacy: Patient data must be handled with the utmost care to protect sensitive information.
- Bias in Data: Biased datasets can lead to skewed results, potentially affecting health outcomes for certain populations.
- Interpretability: Complex machine learning models may act as a black box, making it hard for healthcare professionals to understand how decisions are made.
- Regulatory Compliance: Adhering to healthcare regulations like HIPAA is crucial when working with patient data.
Examples of Successful Applications
Several organizations and technologies have harnessed the power of healthcare datasets for machine learning to achieve remarkable results:
1. IBM Watson Health
IBM Watson Health has showcased the promise of machine learning within oncology. By analyzing massive datasets, Watson can assist oncologists in identifying treatment options that are better suited for individual cancer patients based on their unique pathology.
2. Google's DeepMind
DeepMind has pioneered the use of machine learning in eye disease detection. Their algorithms can analyze eye scans with impressive accuracy, detecting potentially blinding conditions much earlier than conventional methods.
3. Microsoft's Project InnerEye
This project applies machine learning to improve cancer treatment planning by automating the analysis of medical images. By training on clinical images, InnerEye enhances radiation therapy planning, leading to more precise treatments.
The Future of Healthcare with Machine Learning
The prognosis for the future of healthcare, reinforced by healthcare datasets for machine learning, is incredibly promising. Several trends are already emerging:
- Increased Personalization: Treatments will continue to become more tailored to the individual, based on vast amounts of data.
- Telehealth Integration: Remote patient monitoring will become the norm, powered by machine learning algorithms that can analyze data from wearable devices.
- Predictive Healthcare Models: Healthcare providers will lean more towards preventive care, guided by predictive analytics.
- Accelerated Drug Discovery: Machine learning will play a key role in streamlining the drug development process, from identifying potential compounds to predicting clinical trial outcomes.
Final Thoughts
The integration of healthcare datasets for machine learning is redefining the landscape of modern healthcare. While challenges remain in implementing this technology responsibly and effectively, the potentials it holds for improving patient care, enhancing operational efficiencies, and driving innovation in medical research are undeniably significant. As the field continues to advance, it is imperative for stakeholders in the healthcare ecosystem to engage thoughtfully with these tools to create a healthier future for all.
By embracing these revolutionary changes, businesses in the sector—especially those under categories like Home Services, Keys & Locksmiths at keymakr.com—can expand their offerings and enhance their service quality by adopting data-driven insights that machine learning provides.